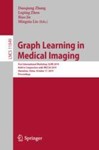
Graph Learning in Medical Imaging
Files
Editors
Daoqiang Zhang, Luping Zhou, Biao Jie, Mingxia Liu
Description
Deep learning has been used to identify Basal Cell Carcinoma (BCC) from pathology images. The traditional patch-based strategy has the problem of integrating patch level information into the whole image level prediction. Also, it is often difficult to obtain sufficient high-quality patch labels such as pixel-wise segmentation masks. Benefiting from the recent development of Graph-CNN (GCN), we propose a new weakly- and semi-supervised GCN architecture to model patch-patch relation and provide patch-aware interpretability. Integrating prior knowledge and structure information, without relying on pixel-wise segmentation labels, our whole image level prediction achieves state-of-art performance with mAP 0.9556 and AUC 0.9502. Further visualization demonstrates that our model is implicitly consistent with the pixel-wise segmentation labels, which indicates our model can identify the region of interests without relying on the pixel-wise labels.
First Page
112
Last Page
119
ISBN
978-3-030-35817-4
Publication Date
11-14-2019
Publisher
Springer
City
Switzerland
Keywords
Basal Cell Carcinoma, Graph-CNN, Pathology
Disciplines
Pathology
Recommended Citation
Wu J., Zhong JX., Chen E.Z., Zhang J., Ye J.J., Yu L. (2019) Weakly- and Semi-supervised Graph CNN for Identifying Basal Cell Carcinoma on Pathological Images. In: Zhang D., Zhou L., Jie B., Liu M. (eds) Graph Learning in Medical Imaging. GLMI 2019. Lecture Notes in Computer Science, vol 11849. Springer, Cham. https://doi.org/10.1007/978-3-030-35817-4_14
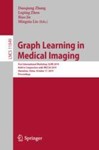